The success of large models can be largely attributed to the existence of Scaling Laws. Researchers have explored the Scaling Laws of transfer learning, examining two metrics: downstream BLEU scores and downstream cross-entropy, and the relationship between the size of the pre-training dataset and the performance of downstream tasks after task-specific fine-tuning. Is cross-entropy loss always a good indicator? BLEU scores appear to follow a logarithmic law more closely. Researchers have provided two guidelines for evaluating the value of pre-training datasets for target downstream tasks. Experimental results show that pre-training has little improvement on BLEU scores, and Scaling Laws apply to the scaling of BLEU scores, which differs from the power-law scaling behavior of cross-entropy and perplexity. The correlation between cross-entropy and BLEU scores is not strong, and the guidelines for pre-training data evaluation offer a method for assessing the value of downstream tasks. The impact of pre-training datasets on task performance depends on the degree of alignment; excessively large pre-training datasets may not yield additional improvements. Scaling Laws can be used to predict improvements in downstream task performance, and whether they can adapt to the scaling of BLEU scores indicates the degree of alignment between pre-training data and specific translation tasks.
Does the Scaling Law for Large Models Also Apply to Downstream Task Performance? Latest Research from Stanford and Google Revealed
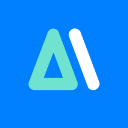
机器之心
This article is from AIbase Daily
Welcome to the [AI Daily] column! This is your daily guide to exploring the world of artificial intelligence. Every day, we present you with hot topics in the AI field, focusing on developers, helping you understand technical trends, and learning about innovative AI product applications.